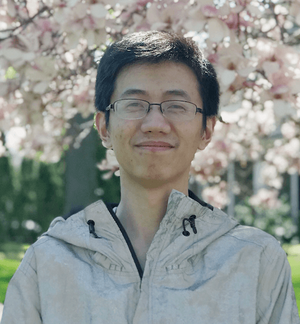
Yuefeng Han
Rutgers University
4:30 PM
127 Hayes-Healy
Colloquium Tea 4:00 - 4:30 PM in 101A Crowley Hall
Subspace Learning for High-dimensional Tensor Data
Motivated by modern scientific research, analysis of tensors (multi-dimensional arrays) has emerged as one of the most important and active areas in modern statistics and data science. High-dimensional tensor data routinely arise in a wide range of applications, such as economics, genetics, microbiome studies, brain imaging, and hyperspectral imaging, due to modern data collection capabilities. In many of these settings, the observed tensors are of high dimension and high order, but the important information may lie in dimension-reduced subspaces induced by various structural conditions. This talk aims to develop new methodologies and theories from a perspective of subspace learning. The talk is divided into three parts. In the first part, we introduce a factor approach for analyzing high dimensional tensor observations, in a form similar to CP tensor decomposition. We develop a new computationally efficient estimation procedure, which includes a warm-start initialization and an iterative concurrent orthogonalization scheme. We show that the iterative algorithm achieves $\epsilon$-accuracy guarantee within $\log\log(1/\epsilon)$ number of iterations. In the second part, we investigate a tensor factor model with a Tucker type low-rank tensor structure. We propose a novel estimation method that is based on the tensor unfolding of lagged cross-product and iterative orthogonal projections of the original dynamic tensors. We also establish computational and statistical guarantees of the proposed method. In the last part, we illustrate tensor factor models using New York city taxi traffic data.
View Poster