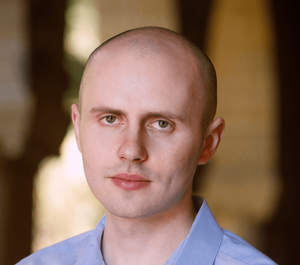
Michael Feldman
Stanford University
3:45 PM
127 Hayes-Healy Center
A tea will precede the event at 3:15 pm in 101A Crowley Hall.
Spectral Properties of Elementwise-Transformed Spiked Matrices
From principal component analysis (PCA) to covariance estimation to factor analysis, spiked matrices of the form Y = X + Z are widely used to model high-dimensional data with latent low-rank structure. Here, X is a low-rank signal matrix and Z is a noise matrix. In this talk, we extend spiked matrix results to the model Y = f(X+Z), where f is a function applied elementwise. This model includes captures forms of missing data, truncated data, unsigned data, logistic PCA, and binomial data with low-rank structure. We find that principal component analysis is powerful for recovering signal under highly nonlinear or discontinuous transformations. Specifically, a phase transition occurs in high dimensions: for signal-to-noise ratios above a sharp threshold---depending on f, the distribution of elements of Z, and the aspect ratio of the data---the principal components of Y (partially) recover those of X. Below this threshold, the principal components of Y are asymptotically orthogonal to the signal. In contrast, in the standard setting where X + Z is observed directly, the analogous phase transition (the Baik-Ben Arous-Péché threshold) depends only on the aspect ratio of the data. Similar phenomena occur with Y, X square and symmetric and Z a (generalized) Wigner matrix.
Click HERE for zoom link (nd.edu access only)
View Poster